Understanding the
Star Schema
What is a Star Schema?
Ideal for a data warehouse or business intelligence, a star schema is a database organizational design that uses one large fact table to hold transactional or measurable data and one or more smaller dimensional tables to keep attributes about the data. It is called a "Star Schema" because the fact table is positioned in the center of the logical diagram and the little dimensional tables branch out to form the star's points.
There is only one fact table in each Star Schema database, and it is the central component of the database. The measurable (or quantifiable) primary data that will be examined, such as financial data, logged performance statistics, or sales records, are listed in the fact table.
It could be a snapshot of historical data up to a specific point in time, or it could be transactional, with rows updated as events happen
How do you operate a star schema?
The fact table contains both numerical values and dimension attribute values. Take into account the following, for instance:
Each row's or data point's numerical value fields are distinct from one another and have no bearing on the information in other rows. Transactional information like the order ID, total amount paid, net profit, order quantity, and exact time may be among them.
In a linked dimensional table, the dimension attribute values—rather than the data—store the foreign key value for each row. The fact table will contain references to this kind of information in several rows.
For example, it might contain the ID of the sales team, a date value, a product ID, or the ID of a branch office. Dimension tables hold the supporting data for the fact table. There is one dimension table in every Star Schema database. Each dimension table will contain additional information about a particular value and be linked to a corresponding dimension value column in the fact table.
An illustration of a star diagram
The employee ID may be used as a key value in the employee dimension table, which may also include other data like the employee's name, gender, address, and phone number.
Information like the product name, production cost, color, and first-to-market date can all be found in a product dimension table.
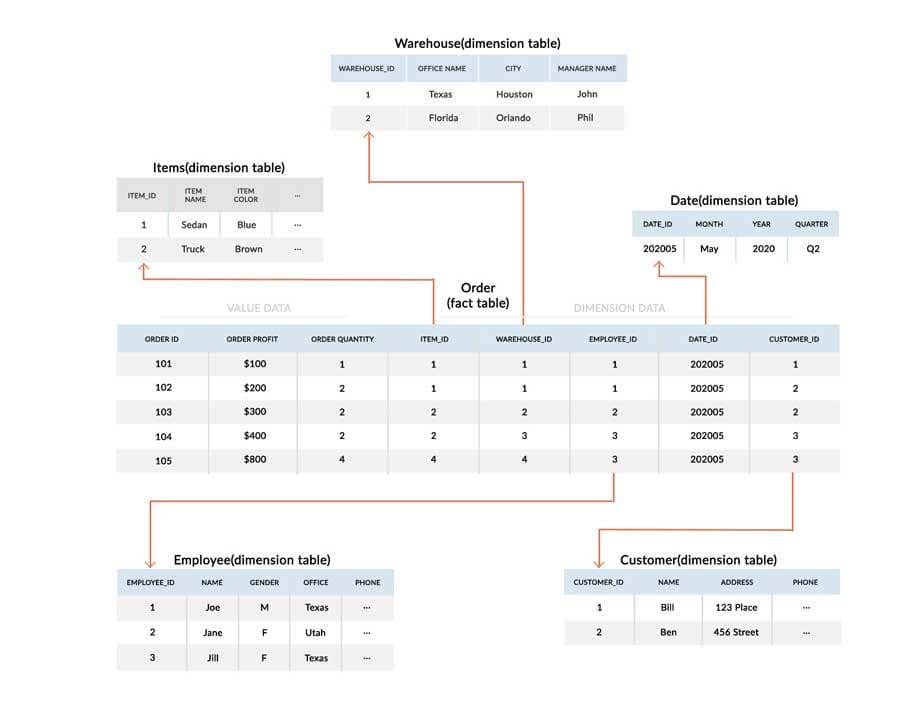
Features of the Star Schema
The following qualities of the Star Schema make it perfect for data warehouse database design:
It creates a denormalized database with quick query response times.
Because of its adaptable architecture, it is easy to upgrade or expand as the database expands and the development cycle moves forward.
It provides a design that is comparable to how end users often perceive and interact with the internet.
Benefits of a Star Schema
Star Schemas are simple to understand and navigate through for both end users and applications. With a well-designed schema, the user can assess large, multidimensional data sets right away.
The main advantages of star schemas in a decision-support setting are as follows:
Less complex questions:
Star Schema's join logic is very simple compared to other join logic that is required to gather data from a well-normalized transactional schema.
Streamlined reasoning for business reporting:
Comparing the Star Schema to a heavily standardized transactional schema, basic business reporting logic—such as "as-of reporting and period-over-period"—is simplified.
Feeding cubes:
All OLAP systems employ the Star Schema to efficiently create OLAP cubes. In reality, most OLAP systems offer a ROLAP mode of operation that allows you to use a Star Schema as a source without having to create a cube structure.
Shortcomings of a Star Schema
Inflexible and lacks data integrity in a highly denormalized Schema state.
Many-to-many relationships aren't supported within business entities.
One-off inserts and updates can result in data anomalies.
Data anomalies can be caused by sporadic inserts and updates.
How Lyftrondata helps
A contemporary data fabric platform solution called Lyftrondata loads the data in Star Schema, offers real-time data access, and lets users query the data using basic ANSI SQL. Businesses can quickly create data pipelines with Lyftrondata, utilizing the power of modern cloud computing with Snowflake and Spark to reduce time to insights by 75%.
Lyftrondata uses straightforward, industry-standard ANSI SQL to make data instantly accessible to analysts, saving engineers the time they would otherwise spend manually creating data pipelines. Pre-built connectors provide a comprehensive search on the data catalog and instantly transmit data to warehouses in normalized, query-ready schemas.
Your all-in-one modern data fabric platform

Data Migration

Data Engineering

Data Sharing

Universal data Model

Data Application

DW Modernization

BI Acceleration

IoT

Governed Data Lake

SaaS BI

Data Virtualization

Data Replication
Plan out your modernization now
Do you want further details on how to handle the most difficult data warehousing problems you're facing? Explore all of our educational and instructive ebooks, case studies, white papers, films, and much more by visiting our resource section.
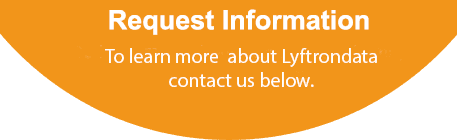
Are you unsure about the best option for setting up your data infrastructure?
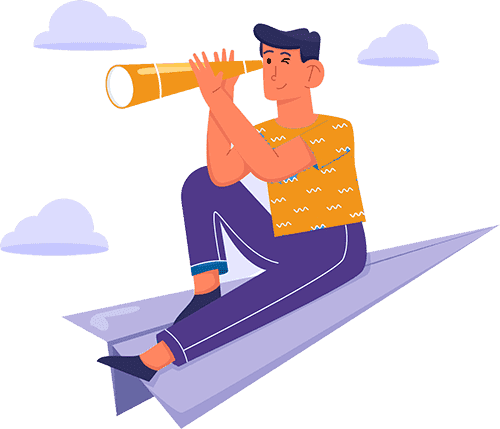