200X Acceleration at
1/10th of the cost
Zero
maintenance
No credit card
required
Zero coding
infrastructure
Multi-level
security
Simplify Qdrant integration in
4 simple steps
Create connections
between Qdrant and targets.
Prepare pipeline
between Qdrant and targets by selecting tables in bulk.
Create a workflow
and schedule it to kickstart the migration.
Share your data
with third-party platforms over API Hub
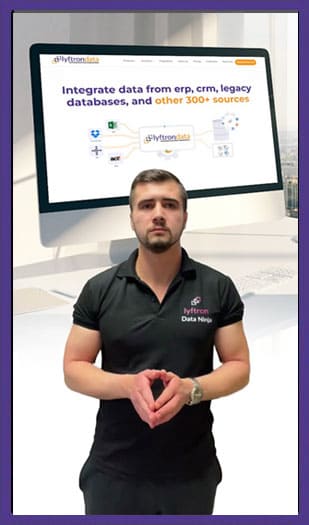
Why choose Lyftrondata for Qdrant Integration?
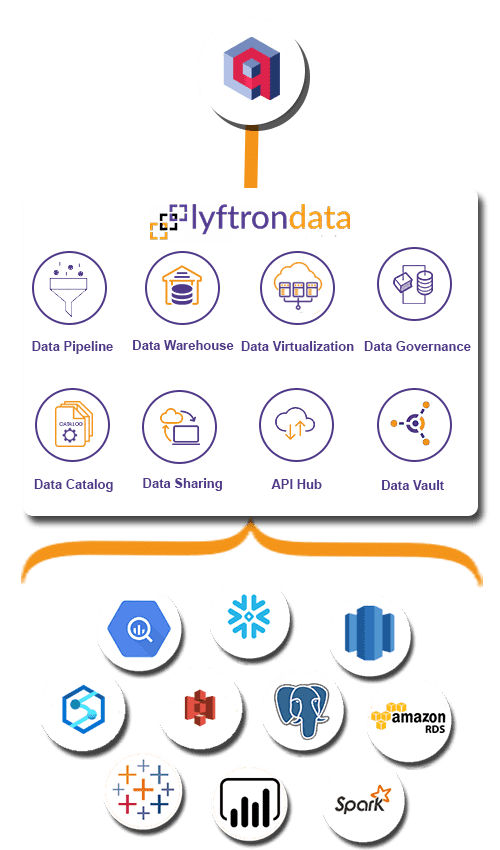
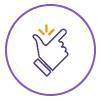
Simplicity
Build your Qdrant pipeline and experience unparalleled data performance with zero training.
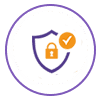
Robust Security
Load your Qdrant data to targets with end-to-end encryption and security.
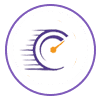
Accelerated ROI
Rely on the cost-effective environment to ensure your drive maximum ROI.
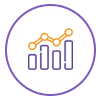
Customer's Metrics
Track the engagement of your customers across different channels like email, website, chat, and more.
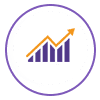
Improved Productivity
Measure the performance of your team and highlight areas of improvement.
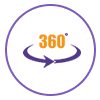
360-degree Customer View
Join different data touch points and deliver personalized customer experience.
Hassle-free Qdrant integration to the platforms of your choice
Migrate your Qdrant data to the leading cloud data warehouses, BI tools, databases or Machine Learning platforms without writing any code.
Hear how Lyftrondata helped accelerate the data journey of our customers
FAQs
What is Qdrant?
Qdrant is an open-source vector database designed for managing and querying high-dimensional vectors, typically used in machine learning and AI applications, especially for tasks like vector search, similarity search, and recommendation systems. Qdrant enables fast and efficient retrieval of vectors, making it ideal for AI-driven applications like image, text, and video search.
What are the features of Qdrant?
Vector Search Engine:
Qdrant is optimized for searching large collections of high-dimensional vectors. It is designed to efficiently perform similarity searches, where the system finds vectors that are closest to a given query vector. This is particularly useful for applications like recommendation engines and content-based search.
High-Performance Indexing:
Qdrant uses advanced indexing techniques such as HNSW (Hierarchical Navigable Small World) graphs, which allow for fast, approximate nearest neighbor (ANN) searches. This indexing method ensures both high accuracy and low latency, even when working with large datasets.
API and Integration-Friendly:
Qdrant provides a simple RESTful API and gRPC interface for interacting with the database, making it easy to integrate into machine learning pipelines and applications. It also supports integration with frameworks such as PyTorch, TensorFlow, and Hugging Face.
Customizable Payloads:
Qdrant allows vectors to be stored with associated metadata, called payloads. This metadata can include any extra information (like document IDs, tags, or categories) and can be used for filtering or ranking results during searches.
What are the shortcomings of Qdrant?
Limited Ecosystem and Features:
Specialized Focus: Qdrant is designed specifically for vector search and similarity search tasks, which limits its capabilities for other data management operations. It lacks the broader set of features you’d find in traditional databases, such as SQL-like querying, complex joins, and relational data handling.
No Support for ACID Transactions: As a vector database, Qdrant does not provide support for ACID transactions (Atomicity, Consistency, Isolation, Durability), which are crucial in many traditional database applications that require strong data consistency guarantees.
No Built-in Analytics or Machine Learning Features:
Lack of Advanced Analytics: Qdrant focuses on vector search and does not have built-in analytical functions, unlike traditional databases that support aggregations, joins, and other analytical features. Users need to rely on external tools to handle more complex analytics tasks.
Limited ML Workflow Integration: While Qdrant can be used with machine learning models for vector search, it doesn’t natively support advanced machine learning operations (e.g., model training, inference) that are integrated within the database. You still need external systems for these tasks.
Real-Time Data Processing:
Limited Real-Time Processing: Qdrant is optimized for vector similarity search but is not designed for real-time data processing or streaming data ingestion. If your application requires real-time updates and searches with constant data changes, Qdrant may struggle to keep up efficiently without significant overhead.
Slow for Frequent Updates: Frequently updating or deleting vectors in a large dataset may introduce performance bottlenecks, as it is not Qdrant's core strength. Managing real-time updates while maintaining search performance can be challenging.
Make smarter decisions and grow your sales with Lyftrondata Qdrant integration
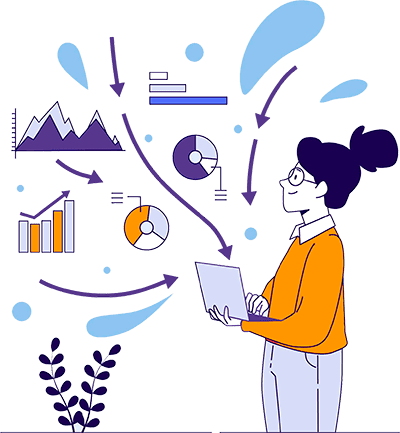