200X Acceleration at
1/10th of the cost
Zero
maintenance
No credit card
required
Zero coding
infrastructure
Multi-level
security
Simplify SnappyData integration in
4 simple steps
Create connections
between SnappyData and targets.
Prepare pipeline
between SnappyData and targets by selecting tables in bulk.
Create a workflow
and schedule it to kickstart the migration.
Share your data
with third-party platforms over API Hub
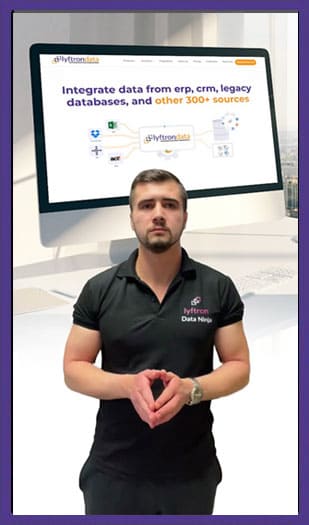
Why choose Lyftrondata for SnappyData Integration?
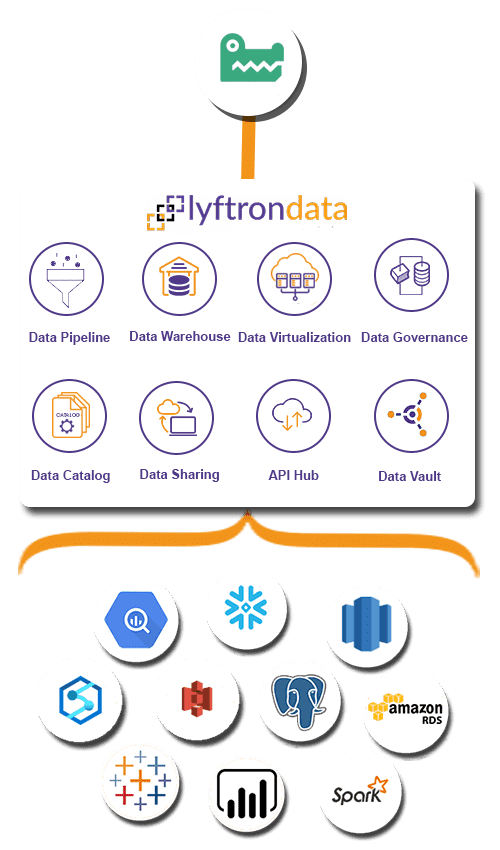
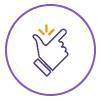
Simplicity
Build your SnappyData pipeline and experience unparalleled data performance with zero training.
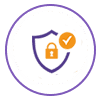
Robust Security
Load your SnappyData data to targets with end-to-end encryption and security.
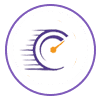
Accelerated ROI
Rely on the cost-effective environment to ensure your drive maximum ROI.
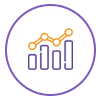
Customer's Metrics
Track the engagement of your customers across different channels like email, website, chat, and more.
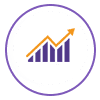
Improved Productivity
Measure the performance of your team and highlight areas of improvement.
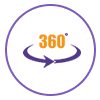
360-degree Customer View
Join different data touch points and deliver personalized customer experience.
Hassle-free SnappyData integration to the platforms of your choice
Migrate your SnappyData data to the leading cloud data warehouses, BI tools, databases or Machine Learning platforms without writing any code.
Hear how Lyftrondata helped accelerate the data journey of our customers
FAQs
What is SnappyData?
The Amazon EMR Integration enables the managed cluster platform known as Amazon Elastic MapReduce, which makes it simple to run big data frameworks like Apache Hadoop and Apache Spark on Amazon Web Services.
What are the features of SnappyData?
Low cost per second: You can take advantage of the low cost per the second service by using the SnappyData Connectors tool.
Amazon EC2 Spot integration: SnappyData ETL offers EC2 spot integration service.
Amazon EC2 Reserved Instance integration: Reserved instance integration with SnappyData Drivers tool.
Elasticity: Amazon Elastic MapReduce, formerly known as the Amazon EMR Integration tool, is a managed cluster platform that makes it easier to run big data frameworks.
What are the shortcomings of SnappyData?
Master node constraints: Any failure of two master nodes simultaneously is not supported by SnappyData Integration.
Cluster recovery issues: Cluster recovery is not possible with the Amazon EMR connector.
Availability Zone failures troubles: Clusters of SnappyData drivers with multiple master nodes are not resilient to Availability Zone failures.
Make smarter decisions and grow your sales with Lyftrondata SnappyData integration
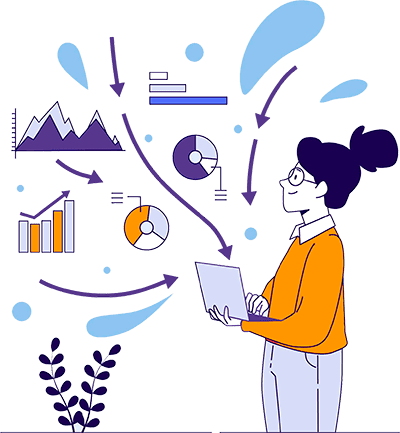