200X Acceleration at
1/10th of the cost
Zero
maintenance
No credit card
required
Zero coding
infrastructure
Multi-level
security
Simplify Vector Database integration in
4 simple steps
Create connections
between Vector Database and targets.
Prepare pipeline
between Vector Database and targets by selecting tables in bulk.
Create a workflow
and schedule it to kickstart the migration.
Share your data
with third-party platforms over API Hub
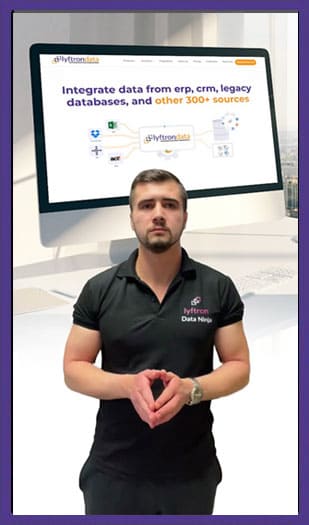
Why choose Lyftrondata for
Vector Database Integration?
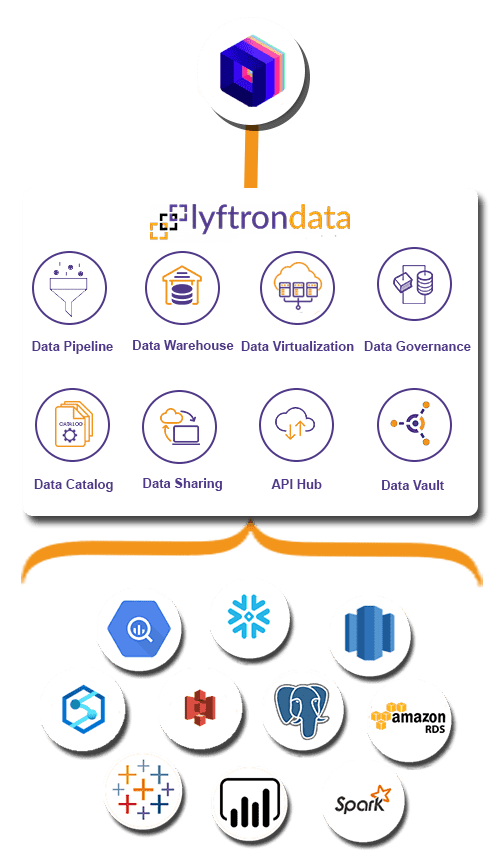
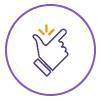
Simplicity
Build your Vector Database pipeline and experience unparalleled data performance with zero training.
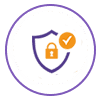
Robust Security
Load your Vector Database data to targets with end-to-end encryption and security.
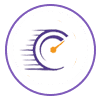
Accelerated ROI
Rely on the cost-effective environment to ensure your drive maximum ROI.
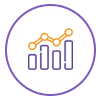
Customer's Metrics
Track the engagement of your customers across different channels like email, website, chat, and more.
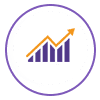
Improved Productivity
Measure the performance of your team and highlight areas of improvement.
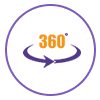
360-degree Customer View
Join different data touch points and deliver personalized customer experience.
Hassle-free Vector Database integration to the platforms of your choice
Migrate your Vector Database data to the leading cloud data warehouses, BI tools, databases or Machine Learning platforms without writing any code.
Hear how Lyftrondata helped accelerate the data journey of our customers
FAQs
What is Vector Database?
Dropbox Integration is a cloud storage platform that enables online file storage and device syncing. You can use Dropbox links to share files and folders with other people without sending large attachments. Dropbox Drivers tool offers a free plan that includes 2 GB of storage.
What are the features of Vector Database?
Cloud storage: Dropbox Integration keeps all your files safe with powerful online cloud storage.
File sharing: Share any file or folder easily with anyone, hassle-free Dropbox ETL.
Productivity tools: Use Dropbox Connectors tools to be more productive in the workplace.
Content collaboration: Dropbox Drivers tool easily collaborates, edit, and share content with team members.
What are the shortcomings of Vector Database?
Limited storage for free users: Users often face storage constraints for the free service of the Dropbox Integration tool.
Organizing files and folders: Dropbox Connectors fall short in the file and folder organization.
Heavy: Dropbox ETL platform is quite heavy to work with.
Sharing content: Sharing content often gets tricky with Dropbox Drivers software.
Make smarter decisions and grow your sales with Lyftrondata Vector Database integration
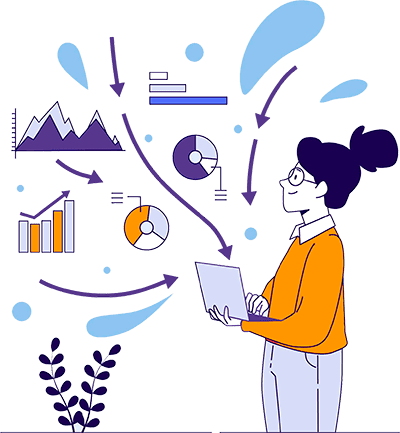